Effective data strategies are essential for leveraging the power of data to drive business growth and informed decision-making. However, the path to a successful data strategy can be challenging. This article explores common reasons for the failure of data strategies and provides insights on how to mitigate these issues.
1. Misalignment with Company Strategy
One of the main reasons for the failure of a data strategy is when it aligns differently from the company’s overall goals.
If your data strategy is not closely tied to your company’s objectives, it can lead to a lack of focus and wasted resources. To avoid this, engaging with key stakeholders, understanding the company’s vision, and designing a data strategy that directly supports the organization’s primary goals is essential. Doing so can ensure that your data strategy is effective and will contribute to your company’s success.
2. You are Trying to do Too Much
Implementing a data strategy can be negatively affected by over-ambition. Attempting to handle too many initiatives simultaneously can stretch resources too thin, leading to a lack of progress and eventual failure. It is critical to prioritize initiatives based on their potential impact and execute them systematically, ensuring that each one is allocated sufficient resources and quality.
In the world of data, it is often true that less is more.
3. Excessive Focus on Use Cases
Defining use cases is essential to data strategy, but focusing solely on them can be limiting. A robust data strategy should take a holistic approach that considers the entire data ecosystem, including data infrastructure, governance, and analytics capabilities. Avoid having tunnel vision and think beyond individual use cases to achieve long-term success.
Data cases tend to be tactical and only sometimes roll up to a whole strategy. A typical example is data repositories. While a data lake/warehouse will undoubtedly power many use cases, designing it only for individual use cases will create silos and inefficiencies. Companies that have designed beyond-use cases have an advantage in the AI race.
4. Lack of Organizational Skill Development
Implementing a data strategy goes beyond just technological advancements. It requires a shift in the organizational skills and culture. It is crucial to address skill gaps, train employees, and promote a data-driven culture to ensure the execution of the strategy. For instance, self-service reporting was unsuccessful for many years because the necessary skills were absent. Organizations must invest in upskilling employees, promoting data literacy, and establishing cross-functional teams to ensure a successful data strategy.
5. Inadequate Technology Infrastructure
Without the right tools and systems, progress can be too slow. If your technology infrastructure is outdated or inadequate, it may prevent you from collecting, storing, governing, and analyzing data. Evaluating your technology landscape, investing in robust data management solutions, and using modern analytics platforms to optimize your data strategy are essential. If your organization is struggling with scattered data, it is vital to have flexible data integration tools to access and model your data rapidly. This problem is particularly severe for large companies with legacy IT and decentralized organizations, where hundreds, if not thousands, of different systems create disparate data.
6. Unclear Goals
Having clear goals and objectives is crucial for the effectiveness of a data strategy. If you lack a clear understanding of what you want to accomplish with your data, you may collect and analyze irrelevant or inconsequential information, which can be counterproductive. Therefore, it is essential to define measurable goals, align them with your company’s strategy, and regularly evaluate progress to ensure your data strategy stays on track.
7. Lack of Stakeholder Buy-In
To successfully implement a data strategy, it is critical to have buy-in and commitment from key stakeholders. To gain their support, engage stakeholders early, involve them in the strategy development process, and communicate the potential benefits. It is essential to use strong business language that focuses on results. Stakeholders don’t care if you are on ETL or ELT; they care about the return on their investments.
Despite recognizing the importance of data, Stakeholders do not commit when they hesitate about the returns.
8. Ignoring Data Privacy and Security
With data becoming increasingly crucial, ensuring data privacy and security is paramount. Neglecting these aspects can result in severe consequences such as reputational damage, legal issues, or data breaches. It is highly recommended to incorporate strict data privacy and security measures into your strategy, comply with industry regulations, and invest in data protection technologies.
Establishing trust in a data organization can take years, but just one data leak can shatter it like a vase falling from a skyscraper.
9. Failure to Iterate and Evolve
Implementing a data strategy just once is not enough to ensure long-term success. To stay ahead in a constantly evolving business landscape, your data strategy must be agile and adaptable to changing business needs and technological advancements. It’s important to regularly evaluate the effectiveness of your plan, learn from both failures and successes and make the necessary adjustments to stay on top of the data-driven game.
10. The Myth of a Single Source of Truth and the Challenges of Data Governance.
For the past 30 years, the idea of a single source of truth has been seen as the solution to all data and analytics-related issues, but it is not a reality. Since people have different perceptions, having a single source of truth is impossible.
While having a single number for public financial reporting is paramount, the realities for a sales executive may differ.
Data governance used to be the latest unicorn that companies chased after under the assumption that it would resolve all data-related problems. However, this task is so enormous that most companies fail. They spend millions of dollars with little or no return on investment.
Many enterprises still need to have a consensus on basic data governance principles before undertaking multimillion-dollar projects to implement it at scale. Try an experiment: ask three colleagues what data governance means to them, and they will likely provide different answers, such as data quality, cataloging, or simply having data owners.
Data governance is a good idea, but companies must decide what level of governance they require and where they need it.
Rather than being broad, it should be more targeted.
Conclusion
A well-executed data strategy can significantly impact organizational success. However, it requires careful planning, alignment with company goals, and adaptability.
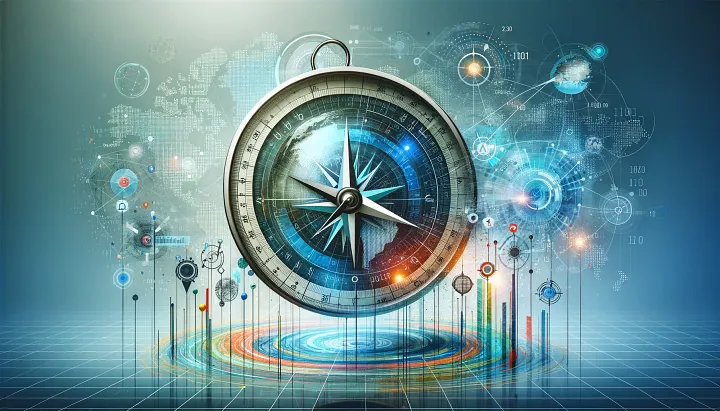
By understanding and addressing these common pitfalls, you can develop a data strategy that drives business growth and innovation while remaining agile in a constantly evolving business landscape.